Self-crashing drone helps improve navigation… really, it does
Researchers at Carnegie Mellon University have taken the bold approach of creating a kamikaze drone to help the development of the technology.
May 15, 2017
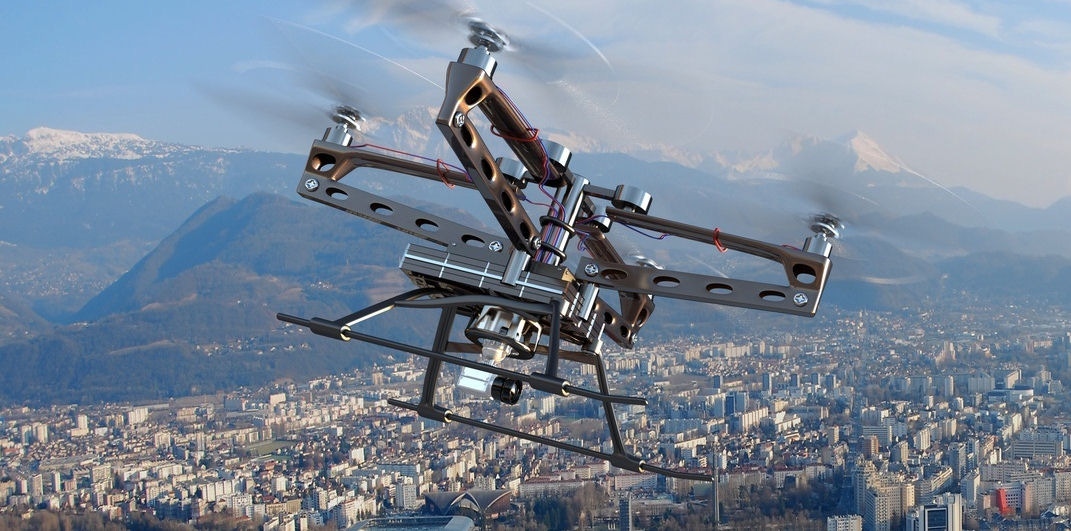
Researchers at Carnegie Mellon University have taken the bold approach of creating a kamikaze drone to help the development of the technology.
Dhiraj Gandhi, Lerrel Pinto and Abhinav Gupta came up with the idea because everyone else was avoiding it, but it is a relatively sound theory. It is easier to understand why not to do something, or how not to do it, when you know understand the build-up and consequences. With this in mind, the team developed a drone which has now be crashed more than 11,500 times.
“The reason most research avoids using large-scale real data is the fear of crashes!” The trio said in a whitepaper. “In this paper, we propose to bite the bullet and collect a dataset of crashes itself! We build a drone whose sole purpose is to crash into objects: it samples naive trajectories and crashes into random objects. We crash our drone 11,500 times to create one of the biggest UAV crash dataset.”
Wandering through the university grounds used to be a pleasant experience, but perhaps not any more at Carnegie Mellon University, as students keep a vigilant eye out for the suicidal drone. The objective is to understand the different ways in which a drone can crash. By collecting all the negative data (the trajectories which crashed) and comparing it alongside the positive data, machine learning algorithms can be used to develop new policies for drone navigation. It’s a simple and blunt way to get to the end result, but you can’t say it isn’t effective.
To assist drones in navigating complex environments a number of developers have been using imitation training to predict how the environment will change. Processing images and using 3D sensors to understand the environment can help the drone understand how it will potentially change, but where does the training data come from? To comprehend certain outcomes, the training data needs to be there to demonstrate it in the first place.
Take for example a human learning to drive. You are risk aware because of what you have seen and experienced. Whether it is TV, training videos, computer games or whatever, you have seen what can potentially happen and therefore are subconsciously looking for potential threats. The negative data being created here will allow drones to do the same thing. In any deep-learning process, the amount of data collected it one of the most important aspects, but also the variety of situations. You need to increase the variety to ensure the drone can react to anything.
Another good example is safety precautions in cars. Cars are purposely crashed to understand what happens. The negative data helps create avoidance so safety features are ultimately improved.
So how good is it? After 11,500 and 40 hours of flying time in a variety of environments, the team claim the drone performs in the range of 2x to 10x better, especially when in more complex environments. It is still not as good as an experienced human pilot, but it is a pretty hefty step forward. The more data which can be collected by the team should lead to further improvements.
But is it better than the traditional way of teaching drones to fly, when obstacles are avoided at all costs:
“By learning how to not fly, we show that even simple strategies easily outperforms depth prediction based methods on a variety of testing environments and is comparable to human control on some environments,” the team claims.
“This work demonstrates: (a) it is possible to collect self-supervised data for navigation at large-scale; (b) such data is crucial for learning how to navigate.”
About the Author
You May Also Like